The 2024 Nobel Prize in Physics has been awarded to two pioneers whose groundbreaking work bridged physics and artificial intelligence, laying the foundation for modern artificial neural networks (ANNs). John J. Hopfield and Geoffrey E. Hinton didn’t just transform AI; they illuminated the profound and surprising connections between physics, biology, and machine learning. Their journey—spanning decades—offers a fascinating look at how ideas from one field can ignite revolutions in another.
In 1982, physicist John J. Hopfield introduced a neural network model that mimicked the brain’s associative memory. His Hopfield Network could store patterns and recall them from incomplete inputs—like recognizing a familiar face from a blurry photograph. The brilliance of Hopfield’s approach lay in applying his expertise in statistical physics, specifically the behavior of spin glasses, a class of disordered magnetic materials.
Hopfield’s network was more than a clever analogy; it was a bridge between physics and computation. He showed that the energy minimization principles governing physical systems could also describe how neural networks find stable states. This idea gave neural networks a formal mathematical foundation and revealed an elegant symmetry: both magnetic systems and neural networks seek to minimize energy, settling into configurations that make the most sense given their constraints.
Geoffrey Hinton, one of the "godfathers" of deep learning, expanded on Hopfield’s ideas in the 1980s. Together with collaborators, Hinton developed the Boltzmann Machine, a probabilistic model that introduced randomness to neural networks. By drawing on the Boltzmann distribution from thermodynamics, Hinton created a system capable of tackling more complex learning problems.
The Boltzmann Machine laid critical groundwork for deep learning. Hinton’s later development of the Restricted Boltzmann Machine (RBM) simplified these models and paved the way for today’s deep learning architectures. These breakthroughs made it possible to train deep, multilayered networks, unlocking the potential for AI to process vast amounts of data and uncover hidden patterns.
The beauty of Hopfield and Hinton’s contributions lies in their interdisciplinary reach. Hopfield’s networks paralleled spin glass systems in physics, where particles settle into stable configurations by minimizing energy. The mathematical elegance extended even further: the Lyapunov function in Hopfield networks resembles the risk minimization strategies of portfolio theory in finance, highlighting the versatility of their ideas.
Hinton’s deep learning innovations found applications across physics, biology, and beyond. In quantum mechanics, these models helped predict quantum phase transitions. In high-energy physics, they enabled particle detection in collider data. Hinton’s work also laid the groundwork for convolutional neural networks (CNNs), key to image recognition systems that now drive facial recognition, autonomous vehicles, and AI-powered medical diagnostics.
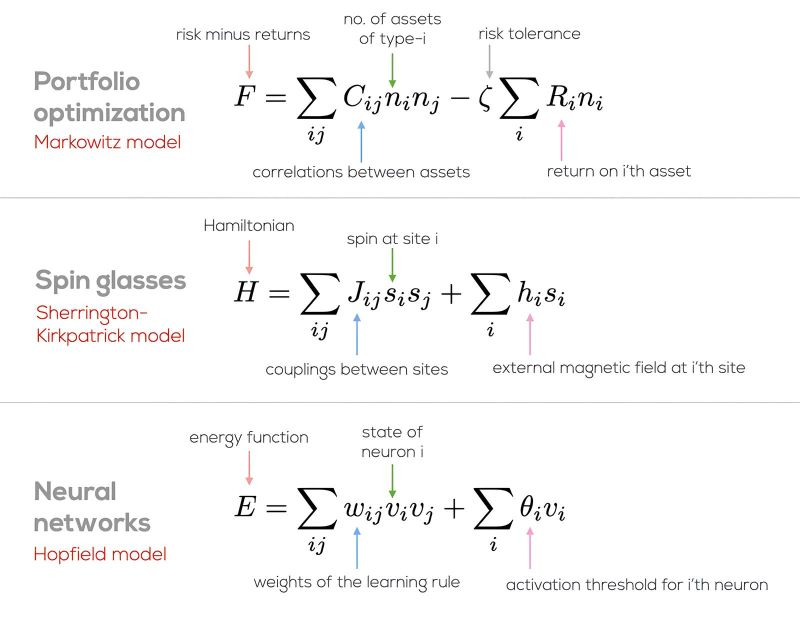
The Nobel Committee’s recognition of Hopfield and Hinton underscores the transformative power of multidisciplinary thinking. Their work demonstrates how concepts from physics can revolutionize AI and, in turn, impact countless industries—from healthcare to transportation.
This award serves as a powerful reminder: progress often comes from connecting ideas across fields. Hopfield and Hinton’s journey exemplifies how breakthroughs arise not just from technical mastery, but from a willingness to explore uncharted territory at the intersections of disciplines. By doing so, they paved the way for technologies like AlphaFold’s protein structure predictions and self-driving cars.
As science and technology become ever more interconnected, the lessons of Hopfield and Hinton’s work resonate more than ever. Their achievements highlight the importance of thinking across boundaries, combining insights from multiple disciplines to tackle complex challenges.
In celebrating their Nobel Prize, we’re not just honoring their past contributions. We’re recognizing the roadmap they’ve provided for future innovators: to see the patterns others miss, to connect the dots between disparate fields, and to push the boundaries of what’s possible.
This article was written in collaboration with LLM based writing assistants.